By Laura Gribble, Data Consultant, GeoPlace.
Addresses are part of our everyday lives. We use them for navigation, deliveries, etc, so they feel ‘simple' or ‘easy’. Unfortunately, there are many different ways to write the same address. These different versions are still functional to humans, but they become unintelligible to query languages without lots of work.
Matching your addresses against AddressBase products allows you to improve the quality of your data by validating your addresses against the definitive record. It enriches your data with classifications and coordinates, enabling deeper analysis including spatial analysis. It provides access to the UPRN and a rich web of linked data which you can mine for insights.
Address matching is a skilled task which requires care in execution or you might find matches being made between different properties, which causes havoc with subsequent services or data analysis.
Address matching practices fall on a spectrum. At either end are the nirvana of 100% instantly automated matches and the dystopia of manually reviewing each and every record individually. Like any spectrum however, there is a large range in between these two extremes.
Data cleansing
Each dataset is unique. You should get to know your data before attempting to match it, for example using data profiling or inspection techniques. This may highlight characteristics or errors in the data which you need to cleanse before matching or be mindful of when matching.
Start by setting your expectations for your data. Here are some starter ideas for this:
- What should be its geographic spread? eg. all UK, just one local authority, etc
- What types of properties should they be? eg. residential, schools, restaurants, etc
- Do you expect of these properties to still exist or will some of them be historic?
Once you have this, you set your matching guideline accordingly. For example, if you should only have current schools, you might exclude matching to historic BLPUs or a school which has been converted into a house. However, bear in mind that a match could provide valuable insight even if it’s not what you expected.
Automated matching
In every file there will be the straightforward, easy matches: building numbers or names plus street plus town plus postcode all match exactly. Depending on the formatting of your file (field names, etc) you should be able to find these in a few minutes. If you are confident in your matching routine then there is no need for a human to look at any of these records. However, a random sample check is still advisable from time to time, especially after making any changes to the routine.
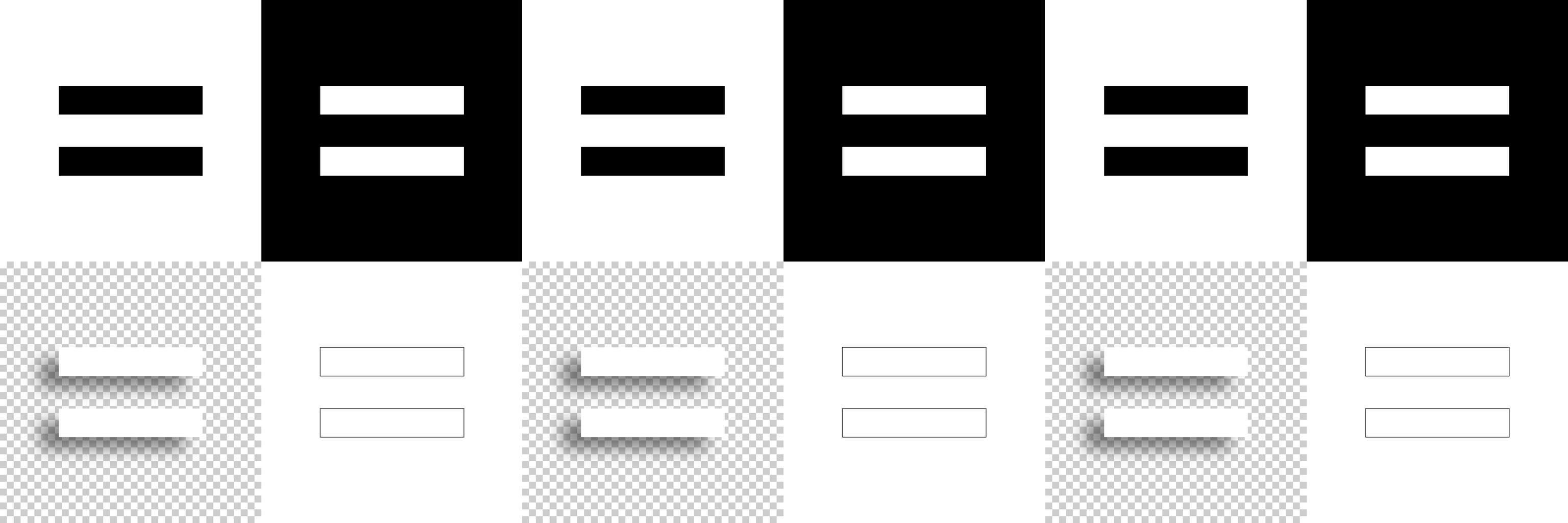
Assisted manual matching
Sometimes automatch routines can be used to make suggestions for ‘most likely’ matches when no exact match can be found. These can then be approved or rejected by a human if they are recognisably the same. For instance, a record with a matching building number but different building or organisation name can be suggested by the routine. As an example, a human would approve ‘W H Smith’s’ being matched to ‘WHSmith' but not to ‘New Look’. You might also allow for common sub-lets, such as a Post Office in a WHSmith.
Bulk review matching
Once the obvious matches have been made via an auto or assisted manual match process, review the data for patterns. If you spot one, it is often possible to write query to find relevant matches for all records with those features. If you are lucky this will match large clusters at a time.
Examples of this matching style include where data is in the wrong fields. You could extract the building number from a street name or search where the street name is in the locality. This is best done with exact matches based on your pattern. If in doubt, review them as with the assisted manual match.
Fully manual matching
Manual searches can be via text or spatial data. Additional confirmatory information is also often required, such as imagery or planning documentation.
This is the most labour-intensive option and requires a skilled analyst to hunt down the correct matches, so it is the method of last resort for most projects. Depending on the data quality it might be possible to make 1000 matches a day or as few as 15.
Fuzzy matching
This section is included as an honourable mention because it is a question we get asked a lot. Please note that we do not recommend using fuzzy matching for most address data matching projects unless you have an experienced analyst, because of the complexities involved.
The general principle here is that this method finds text strings which are similar but not identical. This term has slightly different meanings for different people, however.
You might choose to allow for a set of matching rules, for example common abbreviations or synonyms. The best ones to include will be very dependent on your source data, but possible examples include:
- Rd = road
- Public house = pub
- flat = apartment
- One = 1
Note: Beware of one abbreviation having 2 meanings, eg:
- FL = flat OR floor
- St = street OR saint
Some people choose to automatically ignore punctuation in a text string, but this is not advisable for addresses as removing these characters can change the meaning of address. This is particularly true in Scotland where numbering conventions include the format floor_number/flat_number so if you were to remove the slash you are likely to match to a different property. For example 1/2 should be matched to Flat 2 on the first floor but could be matched to Flat 12 on the ground floor (0/12) or a completely different building all together.
An option is to let a computer determine how similar the two strings are, often expressed as a percentage. Then you can accept any matches over 𝑥 % or have a person review every record between 𝑥 and 𝑦 %. To achieve transparency and confidence in your results, you will need to set 𝑥 and 𝑦 depending on your understanding of how the percentages are generated and attitude to risk (see Understanding false results). Note: common issues with this method include that very short or long addresses can have skewed percentages because each character is relatively important, and there can be confusion over what the comparison is between (eg. each field or the full combined address).
Help, I’m stuck!
If you have any more questions about address matching, we are always happy to talk. Email [email protected] and we can arrange a call to discuss your specific data issues.