By Richard Duffield, Senior Consultant, GeoPlace.
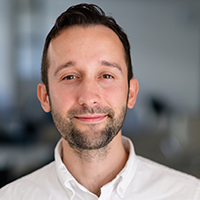
For GeoPlace, one of the great pleasures of working with geographic information is learning so much about life in Great Britain and beyond.
Recently London Fire Brigade (LFB) asked us to work with them on a fire prevention project. This was a chance for us to learn how they keep us safe, and how they are using machine learning, open data and the national address gazetteer to take their approach to the next level. In this blog we’d like to celebrate LFB’s work and show how location intelligence is a vital part of their toolkit.
The Ministry of Housing Communities and Local Government (MHCLG) publishes Energy Performance Certificate (EPC) data. This data shows how energy efficient a property is and how it can be improved. You’ve probably seen a certificate when buying, selling, renting or building a house. Having worked with the data on a previous project we had a hunch that the fire service might find the data useful, so we got in touch.
By happy coincidence, LFB were planning a project - in conjunction with the ASI Data Science fellowship - to investigate how machine learning can predict future fires.
Fire prevention in the UK is a success story and London is no exception. The number of fires in the capital has fallen by around 60 per cent since the start of the millennium.
Apollo Gerolymbos, Head of Data Analytics at London Fire says “Many people just see us as an emergency response service, but we would prefer that people stay safe by never having an emergency in the first place. At London Fire we spend more of our time on prevention work than firefighting so it is important that we target prevention at those people and places where fire is most likely to happen. Last year we visited over 83,000 homes to give free home fire safety advice, with over 68,000 of these visits targeted at people who are statistically considered ‘high risk’”.
Through talking with Apollo and his colleagues we learned that LFB know a lot about fire safety. For example, did you know that the most common cause of fires in London is cooking? Or that the number one cause of fire fatalities is smoking? Other risk factors include electrical items, heaters, gas fires, open fires and candles. Check out the LFB website or the website of your local fire service to learn more. For example, do you perform your nightly “bedtime checks”? Until this project I didn’t – but I do now!
LFB commissioned Tudor Thomas, a data scientist through the ASI Fellowship for a six-week project with two phases. The first was to build an “address catalogue” with details of every building in London and the second was to analyse this data to predict fires.
Apollo adds: “Firefighters collect lots of datapoints at the incidents they attend. Coupled with machine generated data and external datasets we find ourselves to be an information rich organisation. It is our responsibility as analysts to find value and insights in this data to contribute to the safety of Londoners and visitors to the capital.”
To find all addresses within London, LFB used the national address gazetteer which they trust as they use it for call handling and mobilising. For GeoPlace, this is our first machine learning project and we were excited to see how the data would perform.
LFB wanted to collect as much information as possible for each address so they linked the address gazetteer with extra information from:
- Ordnance Survey MasterMap Topography Layer, and
- LIDAR Building heights, and
- Office of National Statistics Index of Multiple Deprivation, and
- Energy Performance Certificates, and
- LFB fire history data
The national address gazetteer provides three ways to link data: the address, the coordinates and the Unique Property Refence Number (UPRN).
LFBs fire history data already contains the Unique Property Reference Number (UPRN) making it easy to link, as does the demographic data.
The national address gazetteer contains a link to MasterMap Topography Layer.
The Energy Performance Certificate data contains a text address for each record. GeoPlace used our address data matching skills to link this data to the UPRN.
The result of this work was a complete address catalogue with a rich set of attributes to analyse.
The chance to learn about predictive analytics came from ASI Fellow Tudor Thomas, a data scientist who did the analysis. Tudor buried himself in the data for six weeks and emerged with a set of findings.
Tudor’s first step was to examine past fires in one London borough. For example, we might think that property tenancy could change the fire risk of a building. This seems logical and is supported by anecdotal evidence. Statistical methods allow us to test these assumptions and add rigour to the approach.
Unfortunately, only weak relationships could be seen, and no single attribute was a strong enough indicator of fire. However, all was not lost. Tudor described how the power of machine learning allows characteristics to be combined to see if together they provide a stronger predictor, and how the UPRN made this possible. By combining characteristics in a predictive model, Tudor could build stronger indicators of risk.
Tudor’s choice of machine learning algorithm also allows us to quantify how useful each attribute is once the analysis is complete. The Energy Performance Certificate data was found to be very useful, particularly as for many addresses it gives a strong predictor when other datasets only give weak predictors.
The next step was to re-train the model on historical data for the whole city to find other properties at risk.
However, doing this does not mean we have gained new insight. What if we are simply showing that fires will happen where fires have already happened? This is of lesser value and would not require the use of sophisticated algorithms.
To avoid this Tudor removed all properties which have suffered from a fire in the past. The remaining high-risk households could not have been highlighted by historical data alone.
Apollo of the London Fire Brigade says “This analysis gives us the potential to target inspections on a household level and to ensure our fire stations are as prepared as possible for the most likely future demand on our service. Without machine learning techniques we cannot make the best use of the intelligence available to us to target risk”. Tudor Thomas, the data scientist, says “The UPRN was the perfect way to link data from multiple sources to the address catalogue. The predictive power of a machine learning model is only as good as the data we put in. To that end, Energy Performance Certificate data was important in predicting where London’s next fire might occur.”
For GeoPlace it has been really exciting to reveal the potential of machine learning with location data.
As Jeff Bezos, CEO of Amazon recently said "The most interesting thing about machine learning is just how horizontal it's going to be. There's not a single category of business or government or anything, really, that can't improve itself."
If you would like to learn more then please get in touch on [email protected].